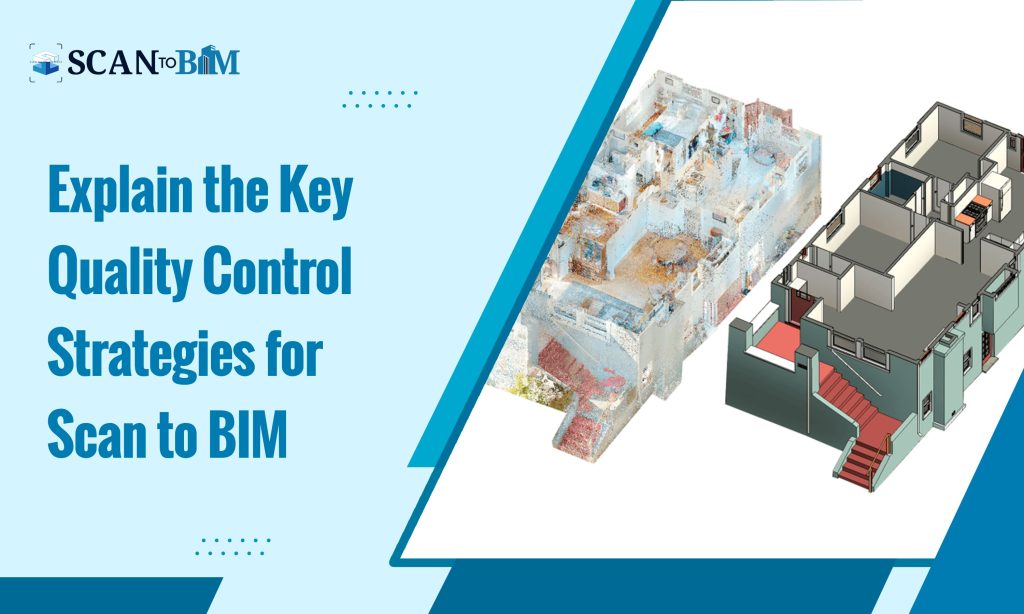
The Scan to BIM process has revolutionized the way we conceptualize, design, and construct the buildings. By digitally capturing the physical attributes of any existing structures through scanning technologies and then converting them into intelligent 3D models. The Scan to BIM Services have demonstrated their efficacy in fostering collaboration, minimizing errors, and optimizing project workflows. However, to assure the accuracy, consistency, and reliability of the resulting BIM models, rigorous quality control techniques are indispensable.
In the below blog, we will delve into the essential strategies for mastering quality control in Scan to BIM processes.
Why Quality Control is Crucial?
Quality control is not merely a checkbox in the Scan to BIM workflow; it is the cornerstone of project success and client satisfaction. Here’s why it’s so crucial:
- Accuracy Assurance: Inaccurate or incomplete BIM models can lead to costly errors during design, construction, and renovation phases. Quality control techniques ensure that BIM models faithfully represent the scanned reality, reducing the likelihood of discrepancies and rework downstream.
- Risk Mitigation: Construction projects are inherently complex, with numerous stakeholders and interdependent workflows. Rigorous quality control mitigates risks associated with design clashes, construction conflicts, and deviations from project specifications, safeguarding project timelines and budgets.
- Data Integrity: BIM models serve as digital twins of physical assets, offering valuable insights for decision-making and facility management. Ensuring data integrity through quality control measures enhances the reliability and usefulness of BIM models throughout the asset lifecycle.
- Regulatory Compliance: Adherence to regulatory standards and building codes is non-negotiable in the AEC industry. Quality control ensures that BIM models meet regulatory requirements, facilitating regulatory approvals and minimising legal liabilities.
- Client Satisfaction: Clients expect accurate, visually compelling representations of their built environment. By delivering high-quality BIM models, AEC professionals enhance client satisfaction, build trust, and foster long-term partnerships.
- Efficiency and Productivity: Detecting and rectifying errors early in the project lifecycle is quite cost-effective than addressing them during construction or occupancy. Quality control streamlines project workflows, enhances productivity, and maximizes efficiency across project phases.
In all, quality control is not an optional add-on but an indispensable component of the Scan to BIM process.
Understanding the Scan to BIM Workflow
Before getting into the depths of quality control techniques, it’s crucial to grasp the Scan to BIM workflow’s intricacies. The process typically involves several stages:
- Scanning: Utilizing laser scanners, photogrammetry, or other scanning technologies to capture the physical attributes of the existing structure.
- Point Cloud Processing: Converting the raw scan data into a point cloud, which represents the precise spatial coordinates of millions of points within the scanned environment.
- Modeling: Using BIM software to transform the point cloud data into intelligent 3D models, incorporating elements such as walls, floors, ceilings, etc.
- Validation and Verification: Assessing the accuracy and completeness of the BIM model in comparison to the scanned reality.
Now that you have an idea about the Scan to BIM processes and why quality control is essential in Scan to BIM, let’s understand the major quality control techniques.
Essential Quality Control Techniques
Pre-Conversion Techniques:
- Data Pre-processing and Clean-up: Before any action can be taken on the point cloud data, it is imperative to prepare the raw scan data for optimal processing. Data pre-processing involves tasks such as splitting, conversions, removing artifacts, and correcting anomalies to enhance data cleanliness and integrity. It also cut downs the size of the data by eliminating the irrelevant data.
- Noise Reduction and Outlier Removal: Noise and outliers in scan data can distort the accuracy of subsequent modeling efforts. Employing noise reduction algorithms and outlier detection techniques like bilateral filtering, spatial depth-pass filter, local density filter, etc., minimizes the data irregularities, ensuring a solid foundation for the conversion process.
While Conversion Techniques:
- Registration and Alignment Validation: During the conversion process, multiple scans are often stitched together to create a comprehensive point cloud. Validating the registration and alignment of these scans is critical to maintaining spatial accuracy as well as coherence within the resulting BIM model. This can be done by identifying common details among overlapping scans.
- Checking Data Validation and Completeness: As data is transformed from point clouds to BIM elements, it is essential to verify the integrity and completeness of the converted data. Automated checks in addition to manual inspections ensure that no critical information is lost or misrepresented during the conversion process. Data density is also checked at this stage as it can lead to a better and more detailed model.
Post-Conversion Techniques:
- Checking for Geometric Accuracy: Validating the geometric accuracy of the BIM model against the ground truth measurements and statistical analyses is fundamental. By quantifying deviations and discrepancies using statistical tools, AEC professionals can identify areas for refinement and optimization, ensuring the alignment with project specifications.
- Semantic Validation by Object Recognition and Classification: Beyond geometric accuracy, semantic validation involves verifying the correctness of object attributes and classifications within the BIM model. Utilizing object recognition algorithms along with classification techniques like machine learning algorithms and computational methods, enables accurate representation and labeling of building elements.
- Comparing BIM Model with Point Cloud Scanned Data: Conducting a comprehensive comparison between the BIM model and the original point cloud data provides deep insights into the fidelity of the model. Discrepancies or inconsistencies between the two datasets highlight areas requiring further scrutiny or refinement.
- Identifying Inconsistencies: Lastly, minute and detailed inspection is essential for identifying inconsistencies or discrepancies within the BIM model. Whether it’s misaligned elements, missing components, or data anomalies, addressing inconsistencies ensures the integrity and usability of the final deliverable.
Conclusion
Quality control is found to be effective Scan to BIM implementation, ensuring the reliability, accuracy, and usability of BIM models for design, construction, and facility management purposes. By integrating the above-mentioned techniques into the Scan to BIM workflow, AEC professionals can mitigate the risks, enhance the project outcomes, and unlock the full potential of digital transformation in the built environment.
Going ahead with quality consciousness and continuous improvement is key to achieving excellence in Scan to BIM processes and delivering superior built environments for generations to come.